1. Introduction
l
Digital breast
tomosynthesis (DBT) is designed to alleviate a tissue overlap problem
occurred in Mammography which is the frequent cause of false positives
(FPs)
l
However, few studies
have been focused on utilizing information between DBT projection
views (PVs) as features for
FP reduction
l
In this paper, mass
features based on inter-view similarity in PVs are proposed to reduce the
FPs
l
The proposed method has
been developed under the following facts:
l
Because PVs are taken in
different angles, FPs induced by the tissue overlap could be observed
differently between PVs, while masses could appear similar (Please see
Fig. 1 (b) and (e))
2. Proposed Inter-view Similarity Features
A.
Proposed inter-view similarity measure
l
By utilizing the
normalized cross-correlation (NCC), inter-view similarity between ROIs on
i-th and j-th
PVs for the given lesion candidate is measured as follows:

where
wi:
the ROI on i-th projection view,
fj
: the subimage on j-th
projection view, s and t: the range of summation taken over the region shared by wi and fj

Fig. 1. Examples of (a) a mass and (d) a FP with
corresponding ROI sets (b) and (e) and inter-view similarities R (c) and
(f), respectively. Note that the ROI sets are sampled from odd PVs
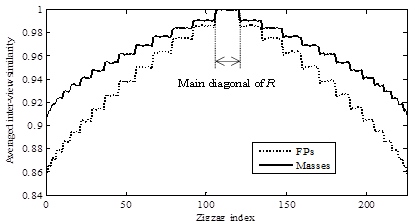
Fig. 2. Zigzag scanned values of averaged inter-view
similarities
B.
Proposed inter-view similarity measure
l
To extract the different
inter-view similarity pattern between masses FPs as shown in Fig. 1 and
Fig. 2, following features are devised
Table 1. Summary of the
proposed inter-view similarity based features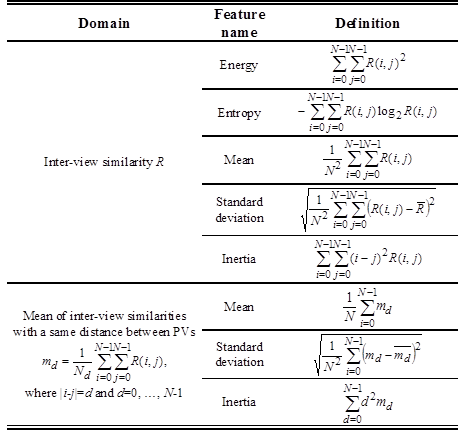
3. Experiments
A.
Experimental setup
l
Dataset: 43 sets of mass
ROIs and 306 sets of FPs are generated by automatic mass detection
l
Classifier: Support Vector
Machine (SVM) with RBF kernel
l
Performance metric: area
under the ROC curves (AUC)
l
50 independent runs of
random partition into training and testing sets
B.
Experimental results
l
The proposed features
show higher AUC with small number of feature dimensions
l
The concatenated feature
(proposed+LBP) achieves an improved AUC. This
indicates the proposed features have complementary information with
existing features
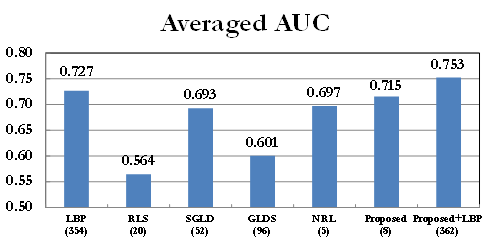
Fig. 3. Comparisons of classification performances in
terms of AUC
4. Conclusions
l
In this paper, we
proposed a new inter-view similarity based features for classifying
masses and FPs, aiming to effectively utilize inter-view information in
PVs
l
Experimental results
show that the proposed features can improve the mass classification performance
in PVs
|