1.
Introduction
l
Computer-aided
Detection (CADe) for breast cancer screening
using mammography
n
To help
radiologists find cancer
n
To help
radiologists avoid missing cancer
l
Main
limitation of current CAD systems is high false-positive (FP) detection
rates
n
Unnecessary
biopsies
n
Financial
expense to the health care system
n
Adding
unnecessary workload to radiologists/pathologists/surgeons
l
We
propose a new and novel approach for the extraction of texture features
for FP reduction purpose
n
Using
Local Binary Pattern (LBP) operation
n
Charactering
regional texture patterns of mass subregions
n
Capturing
spatial structure information of mass
n
Employing
multiresolution analysis
2.
LBP texture feature extraction
2.1.
LBP operation
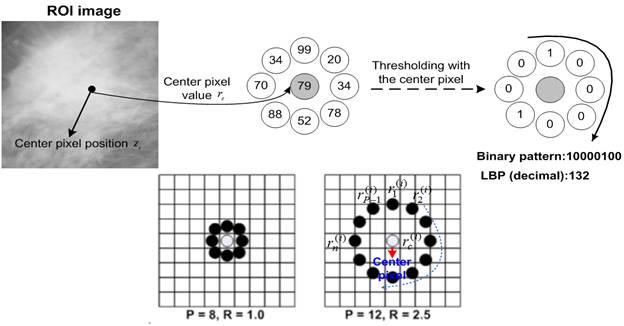
2.2.
Region-of-interest (ROI) partition
l Objective
n To characterize texture patterns
of the masses on a regional level
n To capture spatial structure
(global geometry) of the masses
l Rationale
n A mass ROI is likely to be have
several subregions showing different texture
statistics
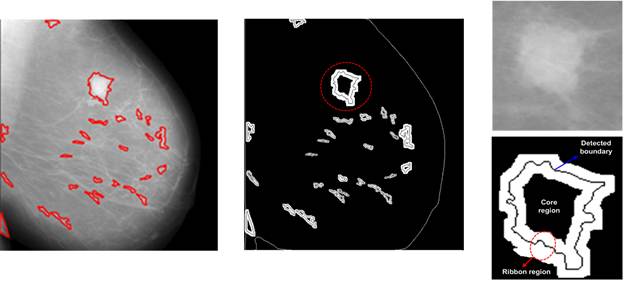
2.3.
Extracting LBP Patterns of Core and Ribbon Regions

2.4.
Multiresolution Analysis
l Exploiting additional
discriminant information
l Using LBP operation for any
quantization of the angular space and for any spatial resolution

2.5.
Hierarchical Encoding Structure
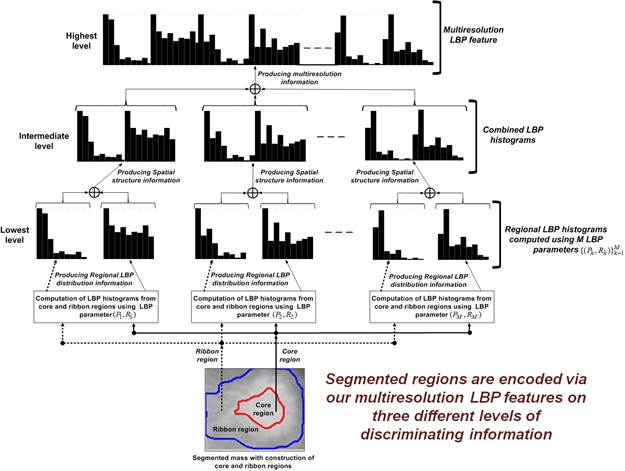
3.
Experiments
3.1.
Experimental setup
l
Mammographic
Image Analysis Society (MIAS) dataset
l
89 case
samples/1,693 ROIs (72 mass ROIs and 1,621 normal ROIs)
l
Support
Vector Machine (SVM) classifier
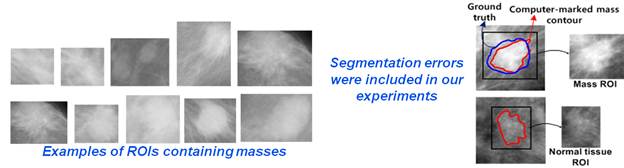
3.2.
Experimental result
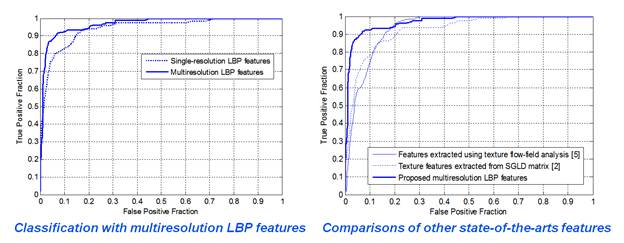
4. Conclusions
l
LBP
texture patterns extracted from both core and ribbon regions are
effective for classification of mass and normal tissues
l
Incorporating
multiresolution analysis is helpful for improving the classification
l
Studies
are underway with a larger and more generalized dataset collected from a large
number of patients undergoing routine mammography screening
|